Forming Queries with Rasters#
Querying the database with rasters is essentially the same as with the other two tables. A primary difference however is they are returned as a rasterio dataset instead of a dataframe.
Grab a whole raster.#
Grabbing whole rasters can be done (albeit with caution) using the from_filter
function.
Note: snowexsql will throw an error if you try to pull more than one dataset at a time. This is because this function is merging tiles together based on the query and if the dataset grids dont match the database throws a cryptic error. So we took the liberty ahead of time.
Try this : To see the error in action, remove the date from the query and run it.
# import in the raster measurements class
from snowexsql.api import RasterMeasurements
from datetime import datetime
from rasterio.plot import show
# Pick a date
dt = datetime(2020, 2, 13)
# Query db filtering to swe on a certain date surveyed by ASO
ds = RasterMeasurements.from_filter(observers='ASO Inc.', date=dt, type='swe')
# Plot it up!
show(ds[0], vmin=0.1, vmax=0.4, cmap='winter')
# Note the resolution!
ds[0].res
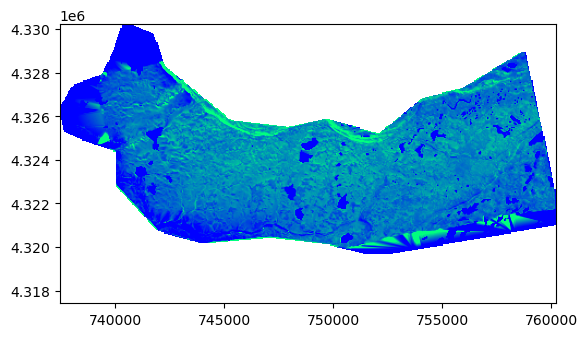
(50.000101089121436, 50.000101089121436)
Let’s get part of raster dataset centered on a point#
More reasonably, we often want chucks of rasters given an point or area of interest. Below is an example of how to do this off of a point.
# Import in the Raster and Layer measurement classes
from snowexsql.api import RasterMeasurements, LayerMeasurements
from datetime import date
from rasterio.plot import show
# Pick a site ID
site_id = '1N3'
df_site = LayerMeasurements.from_filter(site_id=site_id, limit=1)
# Grab available dates
dates = RasterMeasurements.from_unique_entries(["date"], observers='ASO Inc.', type='depth')
dt = dates[0]
# Subset a raster on our buffered point!
ds = RasterMeasurements.from_area(pt=df_site.geometry[0], buffer=100, observers='ASO Inc.', type='depth',
date=dt)
# Plot it up!
show(ds, vmin=0, vmax=1, cmap='winter')
# Note the resolution
print(ds.res)
# Show the site df and available dates.
print(dates)
# Show off the location dataframe
df_site
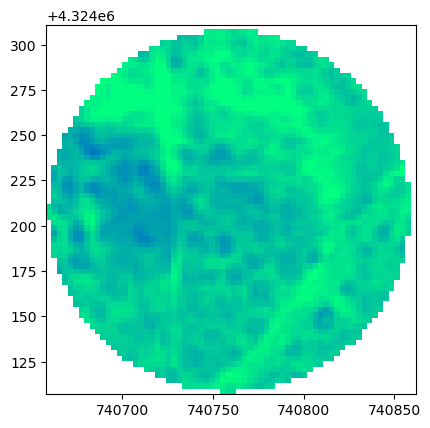
(3.000000000007304, 3.000000000007304)
[datetime.date(2020, 2, 2), datetime.date(2020, 2, 13)]
depth | site_id | pit_id | bottom_depth | comments | sample_a | sample_b | sample_c | value | flags | ... | date | time_created | time_updated | id | doi | date_accessed | instrument | type | units | observers | |
---|---|---|---|---|---|---|---|---|---|---|---|---|---|---|---|---|---|---|---|---|---|
0 | 68.0 | 1N3 | COGM1N3_20200211 | None | None | None | None | None | 45.83 | None | ... | 2020-02-11 | 2024-08-15 20:03:41.020791+00:00 | None | 2414175 | https://doi.org/10.5067/SNMM6NGGKWIT | 2022-06-30 | IS3-SP-11-01F | reflectance | None | Kate Hale |
1 rows × 29 columns
Useful rasterio function - .sample
#
# Demo a useful function from rasterio!
# grab the xy as a tuple e.g. (x,y)
xy = (df_site.geometry[0].x, df_site.geometry[0].y)
# Use the rasterio sample function and the grab the sample
sd = [s[0] for s in ds.sample([xy])][0]
# Print it out nice and neat!
print(f"Lidar snow depth = {sd:0.2f}m")
Lidar snow depth = 0.74m
from snowexsql.api import RasterMeasurements, LayerMeasurements
from shapely.geometry import Polygon
import geopandas as gpd
# Lets form a triangle using site IDs
site_id = ['2S6', '2C2', '8N45']
# Grab the unique locations for these
locations = LayerMeasurements.from_unique_entries(['easting', 'northing'],
site_id=site_id)
# Form a polygon object
triangle = Polygon(locations)
# Query the db for raster data in the triangle
ds = RasterMeasurements.from_area(shp=triangle, observers='ASO Inc.', type='depth',
date=date(2020, 2, 2))
# plot it up!
show(ds, vmin=0, vmax=1, cmap='winter')
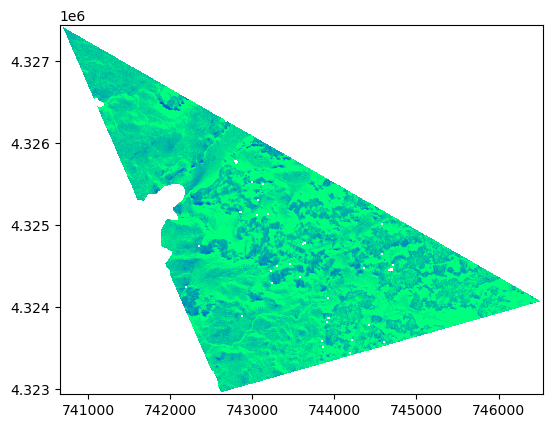
<Axes: >
Recap#
Isolating raster datasets can enable users to build out workflows using only data of interest! No more downloading massive datasets (unless you want to!)
You should know something about
How
RasterMeasurements.from_*
differ fromPointMeasurements.from*
orLayerMeasurements.from*
Raster with too coarse of filtering were error out due to too many datasets.
Rasterio datasets offer a useful sample function for point extraction